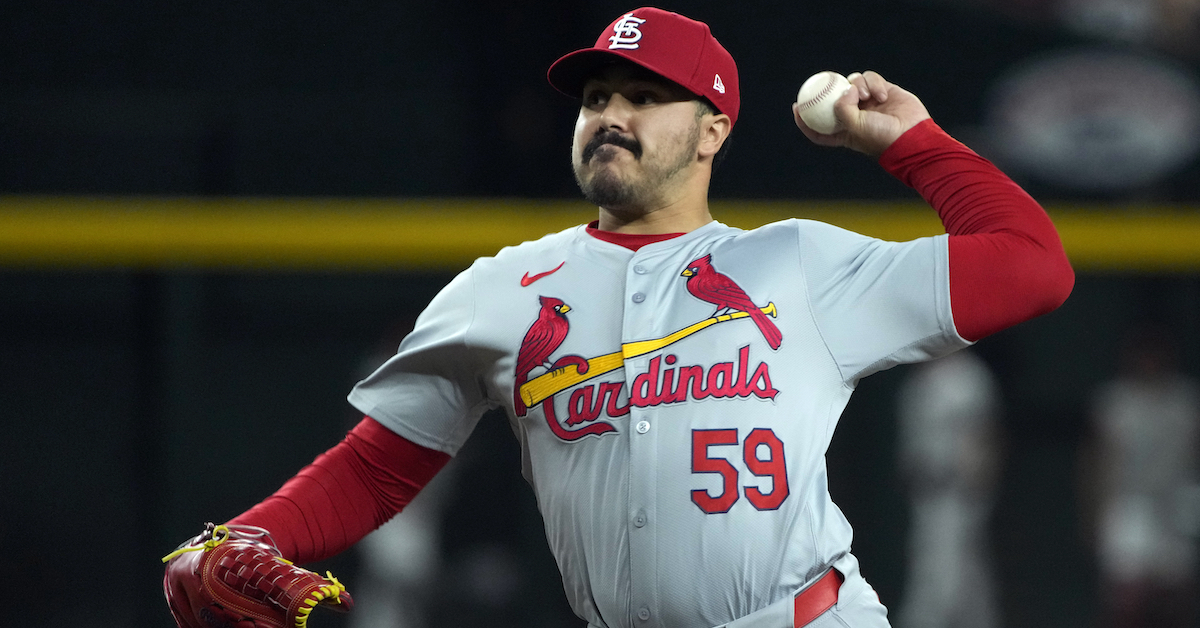
Rick Scuteri-USA TODAY Sports
I’m writing this article for selfish reasons. Every Monday, I chat with FanGraphs readers (come hang out with us! But not next Monday, because it’s a holiday). Four or five times per chat, someone asks a variation of the same question: “Should my team trade this reliever who has been better than expected to a contender for a huge haul?” Four or five times per chat, I say that they should, but that no one would trade with them. So now, I’m trying to put some numbers to it.
The first argument against doing this is fairly simple: Reliever performance doesn’t work that way. To measure this analytically, I took a bunch of recent seasons (2019, 2021, 2022, and 2023) and split them into two. I looked at the correlation between first-half numbers and second-half numbers for every reliever we listed as qualified in the first half of those seasons. I was looking for a simple question: How much can we infer about second-half numbers based on first-half numbers?
The answer, unsurprisingly, is “not very much.” There’s an obvious problem. Relievers simply don’t pitch very many innings. Last year, Jake Bird led all relievers in innings pitched at the All-Star break, with 53.1. Most relievers had meaningfully fewer innings. They didn’t pitch a ton of innings in the second half, either, because that’s just not how relief pitching works. Only 20 relievers threw 70 or more innings last year.
In tiny samples like that, ERA is so noisy that it hardly makes sense. One bad inning, one scorekeeper’s decision, a homer coming with the bases juiced instead of empty, and a good half-season can turn into a bad one. The same is true in reverse. Trying to predict how good a reliever’s second half will go would be wildly difficult even if you knew their true talent level.
That’s not to say there’s no relationship whatsoever. Pitchers with lower ERAs in the first half tend to have lower ERAs in the second half. Pitchers with lower FIPs in the first half tend to have lower ERAs in the second half. Pitchers with lower preseason projected ERAs tend to have lower ERAs in the second half. Pitchers with lower xFIPs in the first half tend to have lower ERAs in the second half. I could keep listing more things if I wanted to, but I’m afraid you might fall asleep reading it.
All of those correlations are significantly significant. Neat! The problem is, they’re also tiny. On their own, any one run-prevention statistic doesn’t explain much variation in second-half run prevention at all. We’re talking 9% of the variation at the most, and mostly much less. Even if I perform some Bad Statistics

and throw all of those into a single multi-variable linear regression, we’re talking 10% of the variation or so.
In plain English, it’s more or less impossible to use information from how a relief pitcher did in the first half to estimate how good their results will be in the second half. Both sides of data are simply too noisy. That roughly tracks with what I expected; “let’s predict the next 30 innings of a reliever’s ERA” is just impossibly hard.
Things get a little better if we’re trying to predict underlying process statistics instead of results. Let’s say, for the sake of argument, that we want to estimate a pitcher’s xFIP in the second half of the season. xFIP is an incomplete statistic, and that’s by design. By forcing a league-average fly ball per home run rate on each pitcher and ignoring actual home runs allowed, it’s eliminating one of the key sources of small-sample variance. I don’t think xFIP is a great statistic in bigger samples; very clearly, some pitchers are homer-prone and others prevent homers well. But in 30 innings of work, homers are just too noisy.
If we’re trying to predict second-half xFIP, first-half xFIP does a good job. It explains roughly 20% of the variance. Preseason ERA projections are nearly as good, checking in around 15%. First-half ERA and FIP don’t do all that well; while we’re now trying to run correlations with a more stable second-half statistic, they themselves have too much first-half noise to be particularly useful. Still, good pitchers in one half are, on average, good in the next half.
Here’s another way of thinking about it. I took the top 10% of relievers in a given year (I’ll use 2021 in this example because it’s up in my spreadsheet right now), as determined by first-half FIP. They produced an aggregate 2.11 FIP and 2.29 ERA. In other words, they were awesome; the average reliever ERA over that span was 4.19. In the second half, that group put up a 3.49 FIP and a 3.36 ERA. Not bad! For the record, their xFIP was 3.76, but now that we’re aggregating a bunch of pitchers together, I feel the sample is probably large enough that I can stop using xFIP.
Those are good numbers! The top 10% of relievers in the first half of the season were actually really good. By comparison, the bottom 10% of qualifying relievers in the first half were putrid: 5.52 FIP, 4.96 ERA. In the second half, they were still quite bad: 5.09 FIP, 5.55 ERA. See? If you’re a good reliever in the first half, you’re likely to be one in the second half too.
My stats-inclined readers, and probably plenty of others as well, are surely screaming at their screens reading this. There’s a huge problem here: selection bias. The relievers who have great first halves are often just great. Great closers like Josh Hader, Edwin Díaz, Emmanuel Clase, and Ryan Pressly showed up on that list of excellent first-half relievers.
This study isn’t really interested in those guys; the question at hand is whether teams can trade their relievers who came out of relative obscurity to dominate the first half and get value back in the deal. It would be absurd to use Hader as evidence in favor of that. So let’s try something else. I took the top 20% of first-half relief performances and filtered out relievers who had been projected to be at least 20% better than league average before the season. That’s about the cutoff for elite relievers, in my mind, and we only want to look for the guys who ascend to prominence with a good half. You could use a different cutoff and probably get marginally different results, but I’m just spitballing here.
The remaining relievers were pretty good! They had a 2.58 FIP and 2.80 ERA in the first half. How’d they do afterward? They pitched to a 4.13 FIP and 3.86 ERA. In other words, they were scarcely better than league average. For completeness’s sake, I looked at how we’d projected these pitchers before the season, and we had them with an aggregate 4.09 ERA.
Again, let’s go to plain English: Relievers who jump from unremarkable to excellent in the first half of a given year tend to be unremarkable again in the second half of the year. Sure, there’s a correlation between good performance in one time period and good performance in the next. But it’s weak, and plenty of it is driven by pitchers who are excellent to begin with. You shouldn’t read too much into a few months’ data, even if the process statistics in that data are excellent.
There’s one other argument I frequently hear around why some non-contending team’s reliever should fetch a big haul in trade: team control. Imagine having five or six years of elite relief work! Sure, maybe the inherent noise in reliever ERA means it might not pan out this year, but with that many bites at the apple, something will surely stick. If you’re looking for a 2024 example of this, think JoJo Romero, who’s been good for two straight years and won’t reach free agency until after the 2026 season. Two and a half years of control! Imagine all the high leverage innings you could get out of him.
How valuable are those future innings? I took an abstracted look at it. My version of the question is this: Given a good relief performance in one year, what should we expect two years down the line? Mathematically speaking, I looked at the top 20 relievers who pitched 50 innings in a given year and then looked at how that group did two years later. I ended up with three three-year pairs: 2017-2019, 2019-2021, and 2021-2023. The shortened 2020 season meant I didn’t want to use data from that year, so I just worked around it.
The results were roughly what I expected. Nearly half the relievers fell out of the sample; in other words, they pitched 50 or more elite innings in year one, then didn’t even complete 50 innings in year three. The ones who did got meaningfully worse. Their ERA increased by roughly 70 points in the gap years. Their FIP increased by 85 points. They went from being among the best relievers in baseball to being merely very good. And again, that counts a lot of elite relievers. Filtering out those names would make the remaining options look meaningfully worse.
When you put this all together, the facts of the matter look pretty straightforward. Why can’t teams flip their overperforming relievers, particularly those with plenty of team control remaining, for incredible prospects? It’s because the other teams in the league correctly assess the future performance of that reliever as wildly volatile and heavily subject to regression. So feel free to keep asking me if your team can turn Andrew Kittredge or Hunter Harvey or Ryan Walker into a Top 100 prospect. But I’ll keep telling you no.
Source
https://blogs.fangraphs.com/no-you-cant-trade-your-newfound-reliever-for-a-shiny-prospect/